- CIO
- CISO
- CDO - Data
- CDO - Digital
- CMO
- AI
- Marketing
- Cybersecurity
- Future of Work
- Leadership
- Career & Talent
- Digital Transformation
- Data
- CEO
- ESG & Sustainability
- Cloud Technology
- Emerging Technologies
- Innovation
- Digital Experiences
- Trends & Insights
- Food for Thought
- Talent
- partner-box
- Collaboration
- Diversity
- Finance
- News
- Women in tech
- Customer Experience
- Events
- partner-soldo
- Culture & Productivity
- Infrastructure
- partner-cmo20
- partner-ifs
- partner-tata
- Digital Skills
- TechGirl
- partner-freshworks
- partner-shure
- partner-trellix
- CMO Strategies
- Service Visionaries
- Wellbeing & Mental Health
- partner-confluent
- Continuous Improvement
- Digital Inclusion
- Community
- Interviews
- partner-8x8
- partner-risk ledger
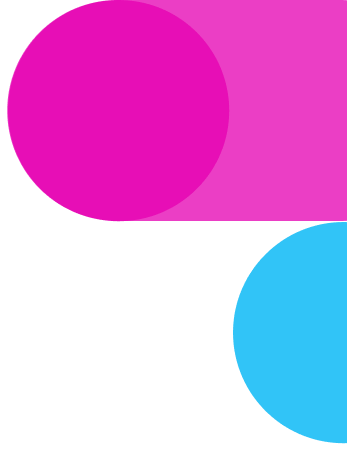
C-suite hot topics & insights
Leading voices in executive leadership
Explore the latest thought leadership, discussions, and insights from our global C-suite community. Discover valuable perspectives from top executives, including CIOs, CDOs, CMOs, CTOs, and CISOs. Stay informed on the latest trends in leadership, innovation, and strategy. Gain actionable strategies to drive your organisation's success forward.
Engage with leading voices in the C-suite community and enhance your understanding of the evolving business landscape.
Meet the HotTopics
contributing editors
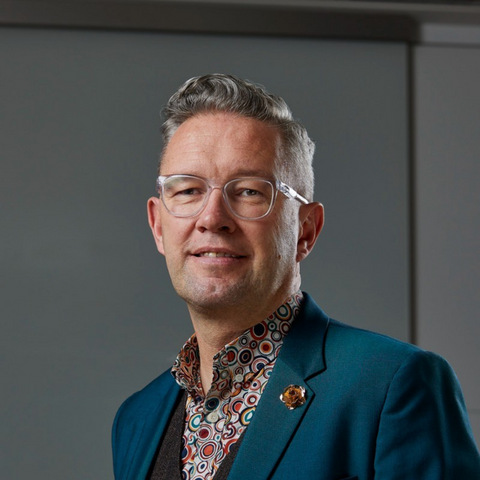
Rich Corbridge
Director General (CDIO), Department for Work and Pensions (DWP)
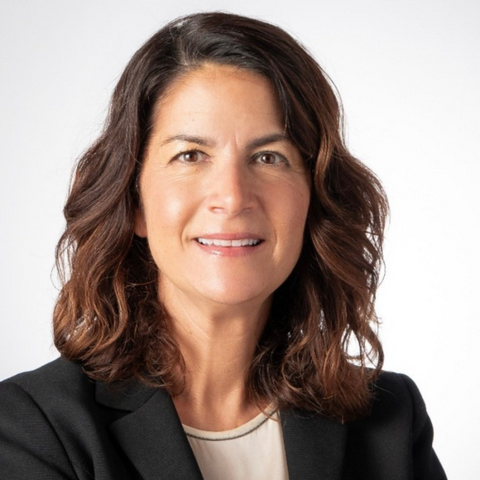
Joanna Pamphilis
CDIO, UniCredit
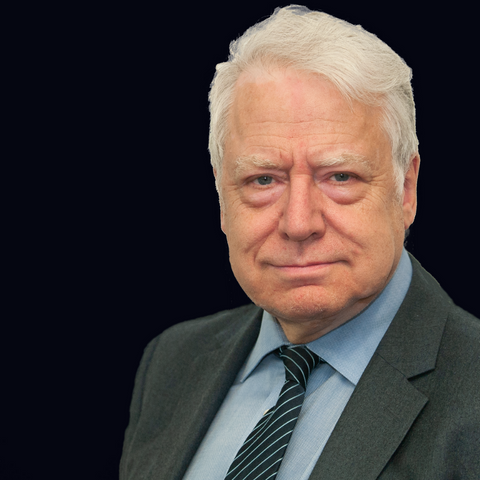
Paul Coby
CIO, Persimmon Homes PLC
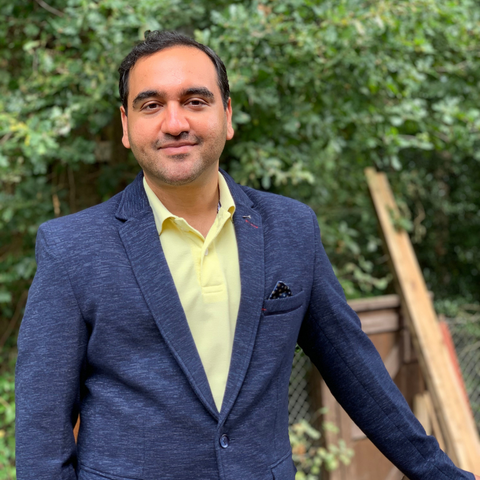
Amitabh Apte
VP, Global CIO, Mars
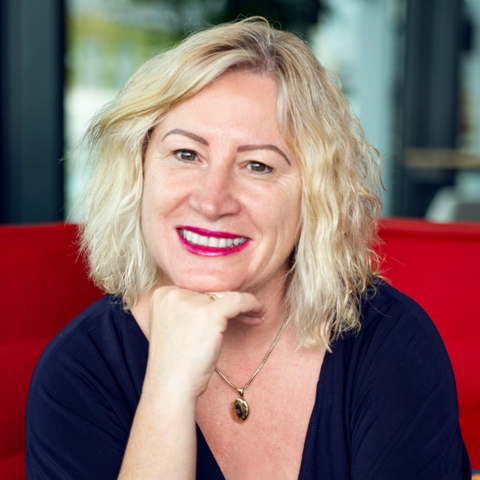
Georgina Owens
CTO, William Hill International
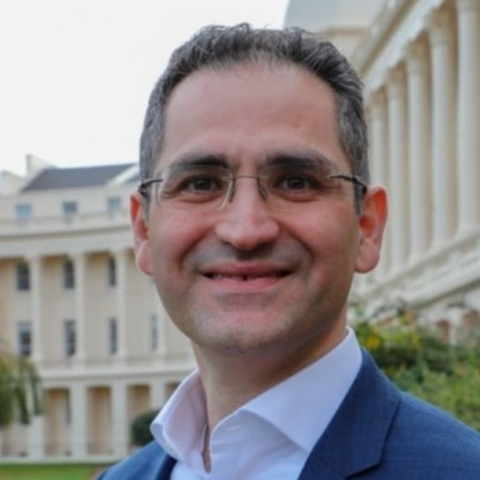
Danny Attias
Chief Digital & Information Officer, London Business School
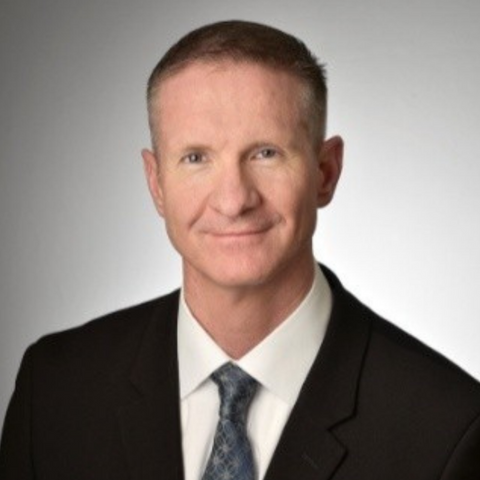
Daniel Bowden
Global CISO, Marsh
.png)
Lee Fulmer
Chairman, Transforming Data Collection Board, Bank of England
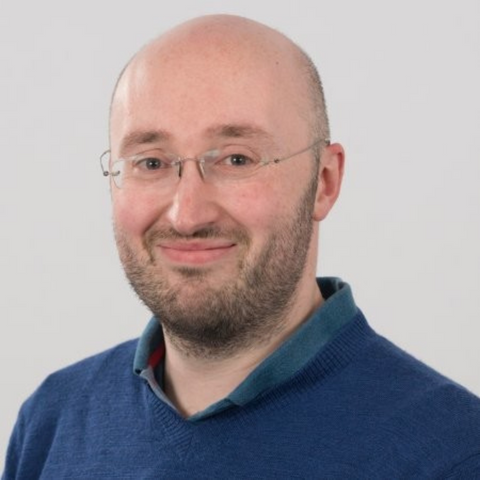
Gerard McGovern
Director, Digital, Tony Blair Institute for Global Change
.png)
Ian Cohen
Chief Product & Information Officer, Acacium Group
.png)
Nafy DIAGNE
Chief Digital and Customer Experience Officer, Free au Sénégal
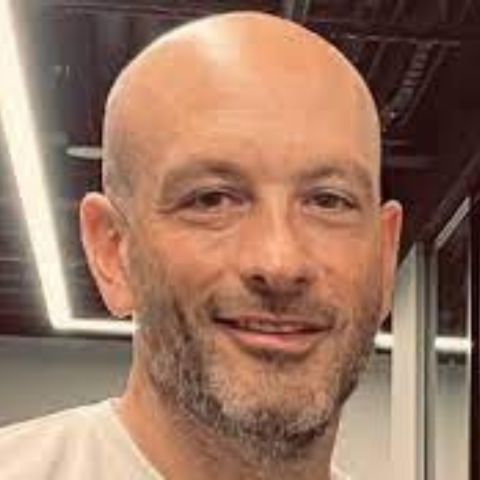
Ash Roots
CDO, University of Exeter ·
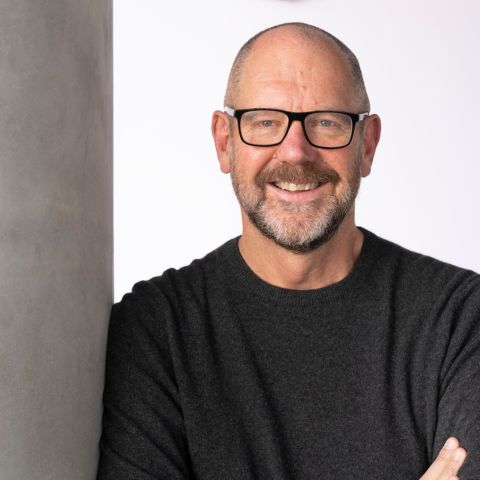
Richard Newsome
CTO, Daemon Solutions
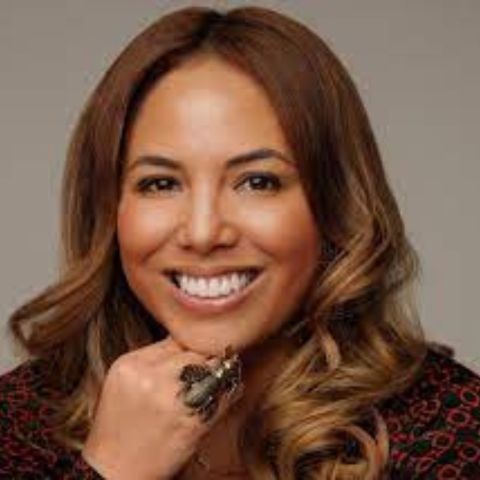
Debbie Ellison
Global Chief Digital Officer, VMLY&R
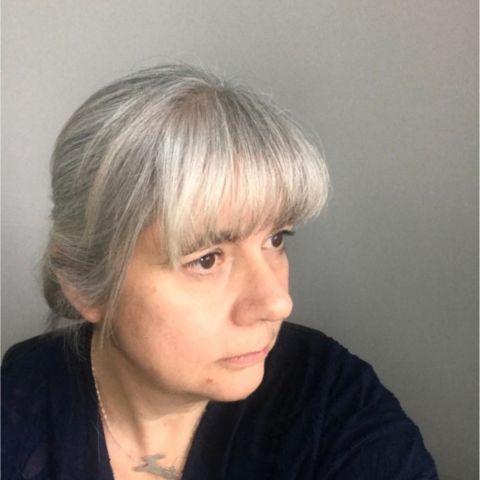
Laura Dawson
Chief Information Officer, The London School of Economics and Political Science (LSE)
.jpg)
Aidan Hancock
CIO, Johnson Matthey
.jpg)
Sandeep Pandey
Group CTO, FWD Insurance
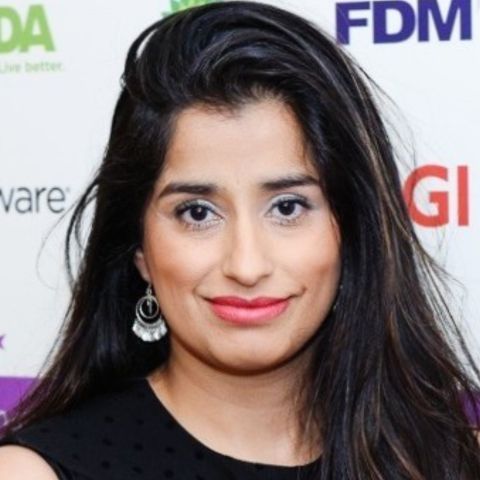
Jots Sehmbi
Chief Information Officer, University of London City
.jpg)
Sarah Needham
Executive Leadership Advisor & Coach, Unique-U Coaching
.jpg)
David Harding
Director, The Harding Method
.jpg)
Emma Smith
Director of Transformation, University of Bath
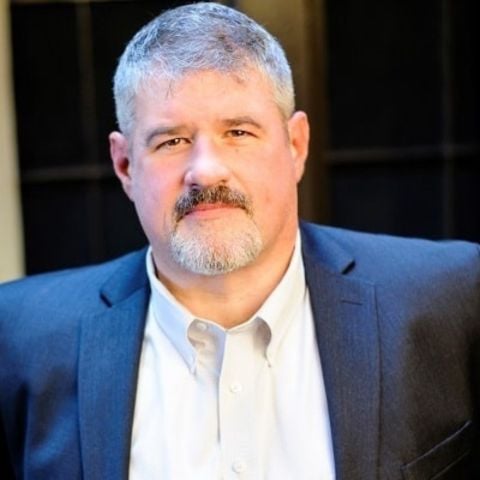