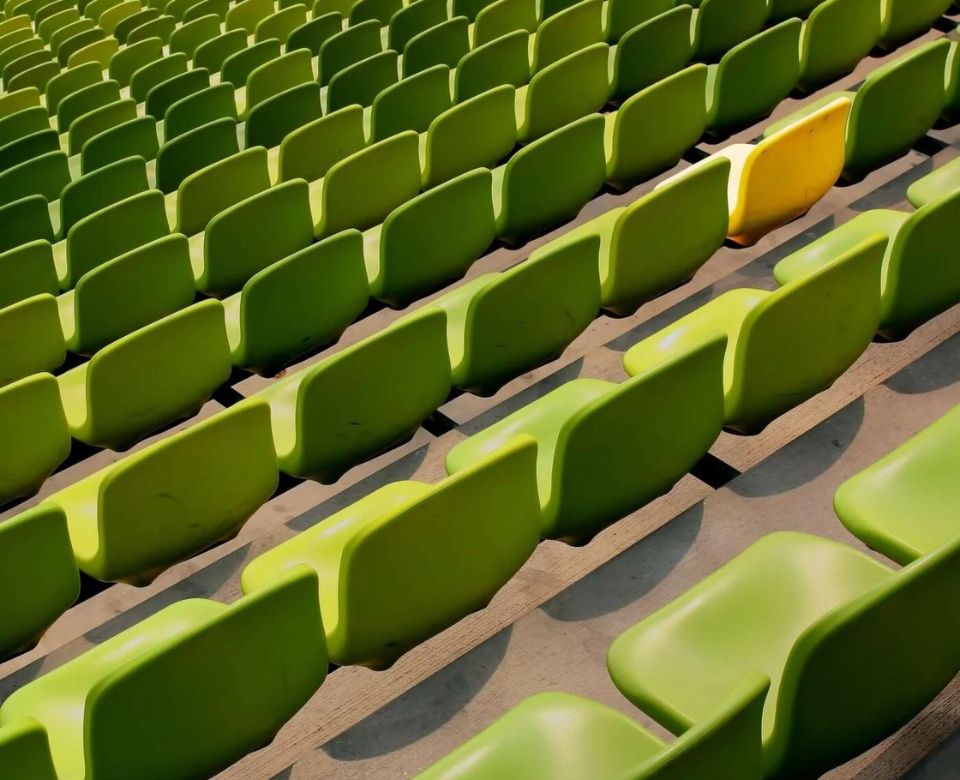
How to spot AI bias
Your new AI solution is only as good as its data, itself reliant on the codes of capture, analysis and conduct of your teams. How can bias wrought AI almost ungovernable?
AI and machine learning are gaining momentum as they revolutionise the way we live and work, with applications ranging from virtual assistants like Siri and Alexa, to cutting-edge facial recognition technology, autonomous vehicles to large language models such as ChatGPT and Bard. However, as AI continues to permeate nearly all aspects of modern society, there is growing concern that the bias with these innovative systems are threatening to derail the promises of the technology.
Bias, naturally and conditionally, found in humans, can have a profound effect on the development and deployment of artificial intelligence (AI) systems. As AI systems are designed and trained by data inputted by human beings, the biases present in the individuals creating these systems can be reflected in their outcomes. This is because algorithms learn from the data provided, so if the data contains biased information, the AI system will learn and perpetuate that bias in its outputs. This can reiterate social and cultural biases present in society, exposing these new technologies as vehicles for injustice as well as innovation.
The consequences of AI bias can be far reaching and serious, therefore. It can lead to unfair treatment of certain groups—and even harm. It is vital that technologists must examine and address the issue of AI bias in a systematic and responsible manner to ensure that technology serves all of us fairly and equitably.
This article delves into the specific challenges posed by AI bias in B2B settings and explores strategies for mitigating these biases.
Overview: how to spot AI bias
- Types of AI bias
- The impact of AI bias
- Strategies to reduce AI bias
- Conclusion
- Learn more: AI governance for data-driven business
Types of AI bias
AI bias can have profound impact on products and services offered by technology companies and B2B organisations, as well as on their business operations more broadly. In order to effectively address this problem , it is essential for companies to understand the types of AI bias.
Demographic bias
The individuals who train machine learning models and algorithms used for decision-making in businesses play a crucial role in shaping the output of AI systems. When these individuals unconsciously bring their own biases into the development and training process, it can result in demographic bias in AI systems. For example if a company’s data used to train a model is predominantly from one particular demographic, such as a certain race or gender, the model may make decisions that unfairly favour or discriminate against other groups. This can result in decisions that are not representative of the wider population and can harm the company's reputation and bottom line.
Algorithmic bias
This refers to systematic errors in decision-making algorithms used by businesses. This can occur due to the way the algorithms are designed, the data used to train them or the interpretation of the results. For example, if a company’s algorithm is trained on data that contains historical gender bias, it may make decisions that perpetuate this bias, such as unfairly favouring male candidates for promotion over females.
Explanation bias
This bias refers to the potential for machine learning models and algorithms to provide biased explanations for their decisions. This can occur when models are trained on data that contains biased assumptions, such credit scoring. If a credit scoring algorithm is trained on data that contains historical bias, such as racial discrimination, it may make biased decisions in granting loans and assigning credit scores. In addition, the model may provide biased explanations for its decisions, such as attributing a lower credit score for an individual in a minority group to their ethnicity or race , rather than their credit score or financial behaviour.
Confirmation bias
This type refers to the tendency of individuals and businesses to seek out and interpret information in a way that confirms their existing beliefs and assumptions. This can occur when decision-makers rely on algorithms and models that reinforce their existing biases, leading to decision-making processes that are not representative of the wider population. For example, when a team is responsible for designing a recommendation algorithm for an e-commerce website it is influenced by their own personal preferences and past experiences. They may design the algorithm to only show products that are similar to what they have purchased in their past, neglecting the preferences of the other users. Therefore this can result in a biased recommendation system that only shows a limited range of products to users, rather than considering their individual preferences.
Adversarial bias
This type of bias refers to the potential for AI systems to be intentionally manipulated or biased by malicious actors. For example in a technology company, an attacker could manipulate the data used to train a machine learning model, leading to biased results. This type is especially concerning in high stakes decision-making scenarios, such as lending or hiring decisions. Also it can result in harm to individuals or groups by the manipulations. For example:
- In 2015 Google Photos mislabelled photos of black people as "gorillas" due to a lack of diversity in the model's training data.
- In 2015, only 11% of Google image search results for "CEO" were women, despite women making up 27% of US CEOs. Also Google's advertising system showed high paying jobs to men more often than women.
- In 2018 Amazon was accused of adversarial bias in several areas such as in hiring, product recommendations and delivery routes.
The impact of AI bias
AI bias can have a wide impact on technology in the B2B world. Here are five ways it can case problems :
- Trust and adoption: people may be less likely to use or trust AI systems if they think they’re biased. This can slow down the progress of AI. For example, if a company’s AI system is seen as not treating women fairly, it may face public criticism and lose customers and employees.
- Unfair decisions: AI systems that are biased can make decisions that are unfair to certain groups of people. This can lead to bad results for these people and harm the company’s reputation. For example, if the AI system is biased against women, it may overlook female candidates for promotions or high level jobs, leading to unequal treatment.
- Legal Issues: AI bias can also lead to legal problems. Companies may be accused of discrimination and may even face lawsuits. For example, if a company’s AI system is seen as biased against disabled people, it may face legal action for not treating them fairly.
- Missed opportunities: AI systems that are biased may overlook good candidates or opportunities, leading to missed chances for the company. For example, If an AI system is biased against minority candidates, it may miss out on talented people from these groups.
- Lower efficiency: AI systems that are biased may make decisions that aren’t as effective leading to lower productivity and higher costs for the company. For example, if the AI system is biased against remote workers, it may overlook good remote workers for jobs, leading to higher costs as the company has to hire more employees to fill these jobs.
Strategies to reduce AI bias
Companies should take proactive steps to prevent bias in their AI systems in order to future-proof their businesses. The below is a non-exhaustive list of the key strategies that the whole company should be utilising, from HR to the technology team(s).
- Train AI systems on diverse data
- Regularly audit AI systems
- Implement fairness monitoring tools
- Incorporate diverse human oversight into decision-making
- Make AI systems transparent and explainable
- Build diverse teams
- Have clear ethical guidelines
- Ensure company-wide bias training for people
Conclusion
Given the continued importance and trust we place in new technologies such as AI, the value of its insights is paramount. But knowing these insights can be tarnished by our own biases and acting to effectively reduce them are two different challenges. It is an inconvenient truth to recognise that, one, AI is still very much reliant on human beings, and two, we ourselves need to learn more about ourselves, our biases and fallacies, if we are to experience the promises of AI and machine learning.
Business leaders are well-positioned to step forward here. Not only is AI and its solutions their responsibility, but from the public’s perspective, the teams that build, adapt and evolve the technologies framing data, machine learning and AI, also require leadership too. That means continued training. It means transparent discussions around the makeup of your teams and the way data is collected and from what sources. And it means having the patience and maturity to see it through to completion, again and again. This is an evolving, never-ending challenge.
Learn more: AI governance for the data-driven business
Watch this roundtable, AI Governance for the Data-Driven Business, featuring
- Mohan Mahadevan, VP, Research, Onfido;
- Dax Grant, CIO, HSBC;
- Tony Sweeney, CIO Asset Finance, Close Brothers;
- Pawan Chawla, CISO, Future Generali India Life Insurance,
View AI Governance for the Data-Driven Business
SUBMIT A COMMENT
RELATED ARTICLES
Join the community
To join the HotTopics Community and gain access to our exclusive content, events and networking opportunities simply fill in the form below.